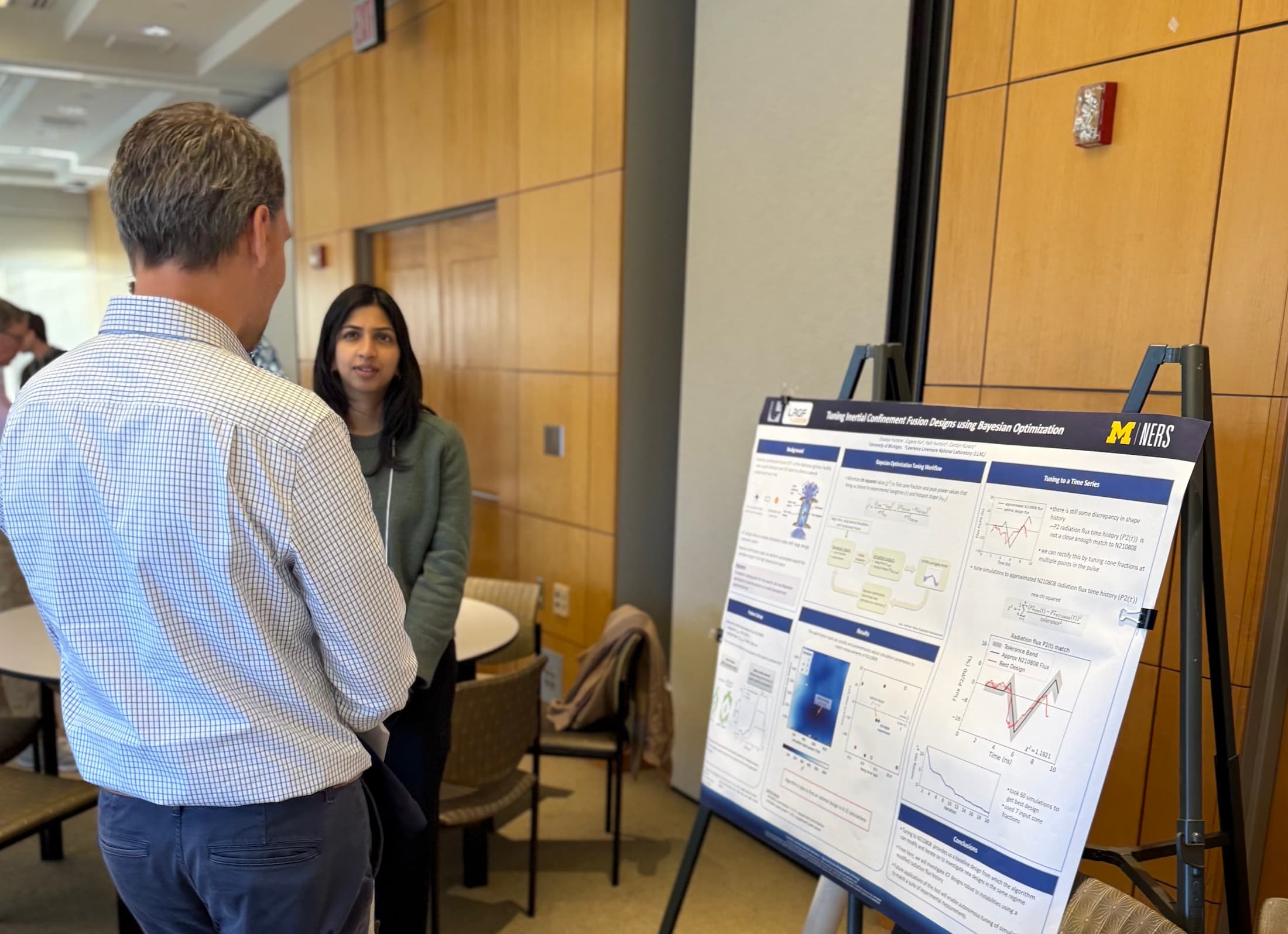
Shailaja Humane wins CoDA 2025 award for fusion design optimization research
The graduate student was recognized for applying Bayesian optimization to inertial confinement fusion simulations.
The graduate student was recognized for applying Bayesian optimization to inertial confinement fusion simulations.
NERS PhD student Shailaja Humane earned (CoDA) in Santa Fe, New Mexico, for her work on applying Bayesian optimization to inertial confinement fusion (ICF) experiments. Under the mentorship of Professor Carolyn Kuranz, Shailaja is developing tools that use machine learning to improve fusion experiment design. Her award-winning poster, “Tuning Inertial Confinement Fusion Designs using Bayesian Optimization,” highlights how autonomous algorithms can speed up and refine the tuning of simulations to better match experimental results—reducing reliance on manual, time-intensive methods. In this Q+A, she reflects on her research, shares insights from the N210808 experiment at the National Ignition Facility, and discusses what the recognition at CoDA means for her and the future of ICF design.
Can you explain in simple terms how Bayesian optimization improves the design process for ICF experiments?
The design process for inertial confinement fusion experiments is complex, usually done by teams of scientists that use multi-physics codes to simulate their experiment designs. There are dozens of parameter choices for these designs, which scientists often hand-tune. Bayesian optimization tools provide an opportunity to quickly and autonomously search through this large parameter space for candidate designs. The optimization algorithm tries to find the best design by testing multiple simulations and eventually converging on an optimum simulation. It balances trying new possibilities (exploration) and improving on what works best (exploitation) to quickly zoom in on the optimal choice.
What insights did your research provide about the N210808 experiment, and how do they contribute to future ICF work?
My work was able to demonstrate autonomous tuning of simulation inputs to match a few of the N210808 experimental measurements. This is something that designers often do by hand, and the success of this research demonstrated a new application for this Bayesian optimization tool. We’re excited to use this tool to tune simulations to match a suite of experimental measurements. Hopefully, this can pave the way for scientists to integrate this tool into their ICF design work.
Were there any surprising results or challenges you encountered during your research?
One challenge we ran into was the algorithm’s sensitivity to the tolerances or uncertainty associated with the experimental measurements. Fine-tuning these tolerances gave me valuable insight into how the algorithm chooses its designs. How well you frame the problem is a huge contributor to the algorithm’s performance.
What was your experience like at CoDA 2025, and how did the poster session go?
This was my first time at CoDA, and my first time presenting my work at a conference that was more data science focused rather than plasma physics or fusion focused. It was extremely valuable to speak with other scientists using similar tools for a variety of science applications. The poster session presented a unique challenge of presenting my work in under 5 minutes. Given that most of the audience wasn’t familiar with inertial confinement fusion physics, this time constraint was especially difficult. I was happy to have this challenge, though. Science communication skills are important and this was a great opportunity to hone those skills.
What does winning this award mean to you, and what are your next steps for this project?
It’s a nice recognition of the effort that went into this research, and I appreciated the opportunity to share my work with experts in data science. This project has been a great learning experience, and I’m excited to continue building on it. We’ll use the N210808 tuned design as a baseline to investigate new designs in the same regime. We also plan to apply the Bayesian optimization tool to tune to broader sets of experimental measurements.
Anything else you’d like to add?
I’m thankful for the advice and support of my mentors, Eugene Kur, and Kelli Humbird, and of my advisor, Carolyn Kuranz. Without them, this work would not have been possible.